Research overview
A physiotherapist or a dance partner intuitively understands their counterpart’s movements. How can we give a robot a similar intuition? This requires understanding the human movement controller and abstracting it in a mathematical form. To shed new light on this problem, our research aims at bridging the fields of biomechanics and motor neuroscience, where we use control and robotic theories to analyze the body and the brain in a unified framework. In return, this holistic theoretical framework informs the design and control of advanced bio-robotic systems.
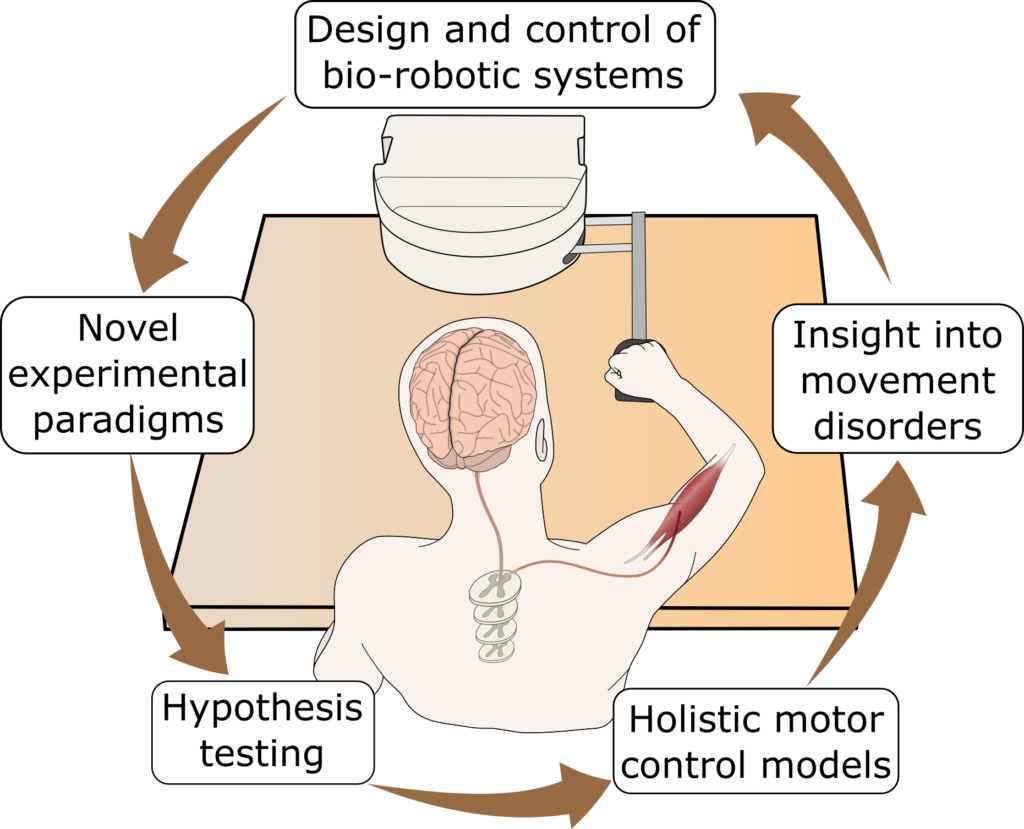
Human-aware control of robots
Current state-of-the-art bio-robots (e.g., assistive exoskeletons or rehabilitation robots) are hard-coded to perform specific actions after detecting the user’s intent. This control paradigm is inherently limiting, as humans inevitably adapt their behavior in response to the interaction with the robot in the short and long timescales. Existing robots are unaware of the ever-changing biological states of the user. Our human-aware controllers encode the knowledge about how humans control and adapt their movements in new environments. Our research aim is to embed these models in robots’ controllers to bring about a paradigm shift in how we build bio-robots—robots that can infer the user’s behavior, capabilities, and limitations, to accordingly respond, adapt, correct, or assist.
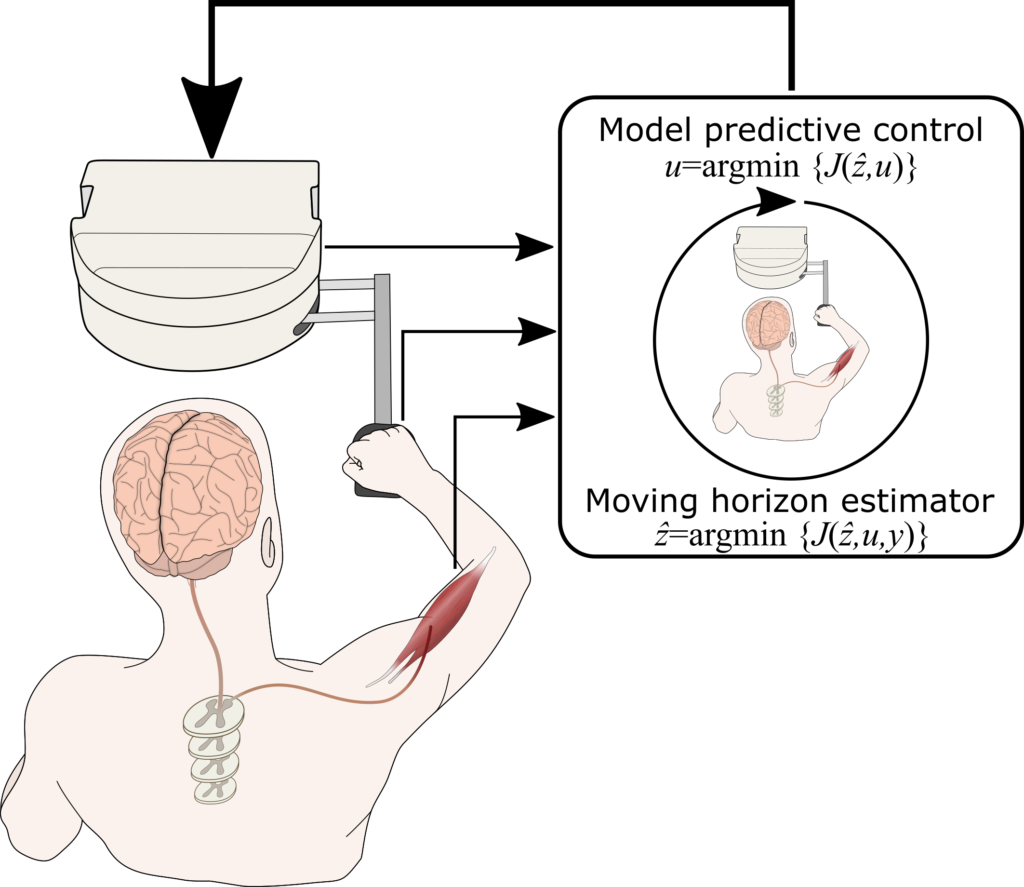
Neuromuscular mechanics of human movements
The sensorimotor apparatus that makes humans so dexterous is vastly complex. Even mundane human movements such as lifting a finger involve multi-scale phenomena ranging from mechanical interactions between protein molecules in muscle fibers to the dynamics of networks of neurons to Newtonian mechanics of the limbs. Our research philosophy is that the complexities of the human musculoskeletal system and its neural control mechanism are tightly interrelated, and one cannot be understood without considering the other. However, the sheer size of the problem has led to the formation of separate research fields that study the body (biomechanics) and the brain (neuroscience) in isolation. Our research aims to provide new perspectives on human movement control by bridging the borderline between the fields of biomechanics and computational neuroscience.
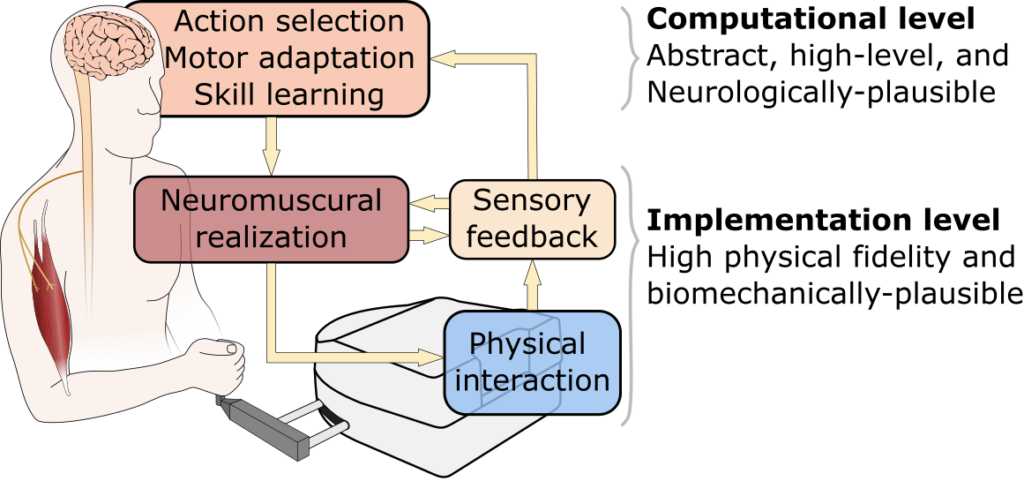
In our work, we aim to combine biomechanically plausible models of human movements with abstract neuroscientific theories to present multi-scale holistic models for human neuromuscular control. These holistic motor control models will present answers to both why- and how-questions in a unified framework. Low-level details, such as the distinction between reflex-based and cortical contributions to muscle activities, and high-level “computational” aspects, such as action selection and skill learning, can be studied together in this framework. In return, the model validation process will inspire new testable hypotheses and experimental paradigms to understand human movements better. More concretely, at the computational level, our current models refute the separation of the brain (as an information processor) from the body (the plant to be controlled) in movement control. Instead, it suggests that an observed action is the autonomous progression of a closed-loop-stable dynamical system (that includes the neural system, the body, and the environment) towards its dynamical attractor. The experimental data, whether rejecting or supporting our hypothesis, will refine our models, which then give rise to new insights and testable hypotheses.
Precision rehabilitation engineering
A prominent technology in rehabilitation engineering is the use of robots to administer post-stroke exercises. This clinical practice, however, has been slow to progress despite its virtues, mostly because we do not know how a patient’s neuromuscular system responds to the intervention. Recent intelligent stroke rehabilitation robots can estimate a patient’s muscular activities during the exercise, thereby allowing the robot to optimally adapt to assist or resist the patient’s motion. Despite their abilities, current rehabilitation robots are still oblivious to the neuromuscular constraints of stroke survivors; electrophysiological studies indicate that patients lose part of their repertoire of neuromuscular modules for the brain to produce movement. In this research, we employ holistic motor control models in the robot’s controller to identify the patient’s neuromuscular constraints, and design exercise paradigms to push the patient out of their “neuromuscular comfort zone” to accelerate relearning of the lost motor function. Because of the heterogeneity of stroke survivors, “precision rehabilitation” is necessary—we need patient-specific exercises. Precision rehab necessities a realistic human movement model in the robot’s control algorithms.
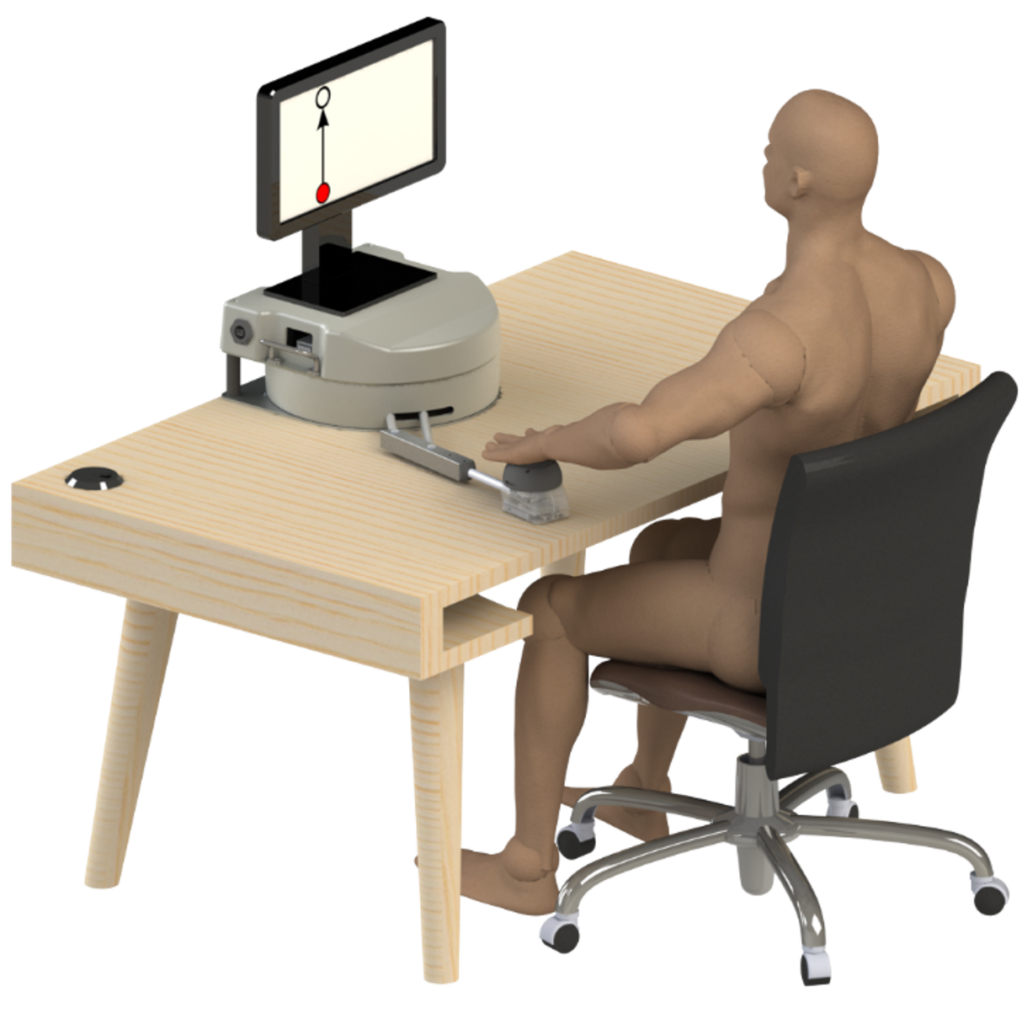